Project title: ADVANCED METHODS OF QUANTIZATION, COMPRESSION AND LEARNING IN ARTIFICIAL INTELLIGENCE
Acronym: Com-in-AI
PI: Dr Zoran Perić, full professor, Faculty of Electronic Engineering, University of Niš
SRO’s: Faculty of Electronic Engineering, University of Niš; Faculty of Science, Technology and Medicine, University of Luxembourg
AI algorithms have been used for decades to successfully solve the most challenging problems, and their impact on humans and society is constantly growing. Due to their complexity, AI algorithms require complex software implementation and significant hardware resources, while consuming a large amount of energy, which limits their application. In order to enable wider application of AI algorithms and implementation on portable devices and terminals, extensive research has been conducted in recent years. A particularly topical direction of research is the application of quantization and compression in deep learning in order to reduce the complexity of algorithms. Although certain results have been achieved, the influence of quantization and compression on the accuracy of AI algorithms has not yet been sufficiently explored.
Com-in-AI will increase the efficiency of deep learning methods and algorithms, with an emphasis on the development of innovative methods of compression and quantization of deep neural network parameters, with a view to enabling digital representation of these parameters using a significantly smaller number of bits while preserving a high accuracy of the neural network. This will result in a reduction in software implementation complexity, execution complexity and required hardware resources, and enable faster execution of AI algorithms.
PROJECT OBJECTIVE: The goal is to digitally represent the parameters of deep neural networks with a significantly smaller number of bits (2-6 bits instead of the usual 32) while preserving the accuracy of the neural network.
METHODOLOGY: Deep learning algorithms, clustering and classification algorithms, algorithms for detection of regularities in data will be used, and statistical analysis and modelling of their parameters will be performed.
EXPECTED RESULTS: The project will develop methods to reduce the complexity of AI algorithms and the required hardware resources using advanced quantization and compression techniques and team expertise in the field.
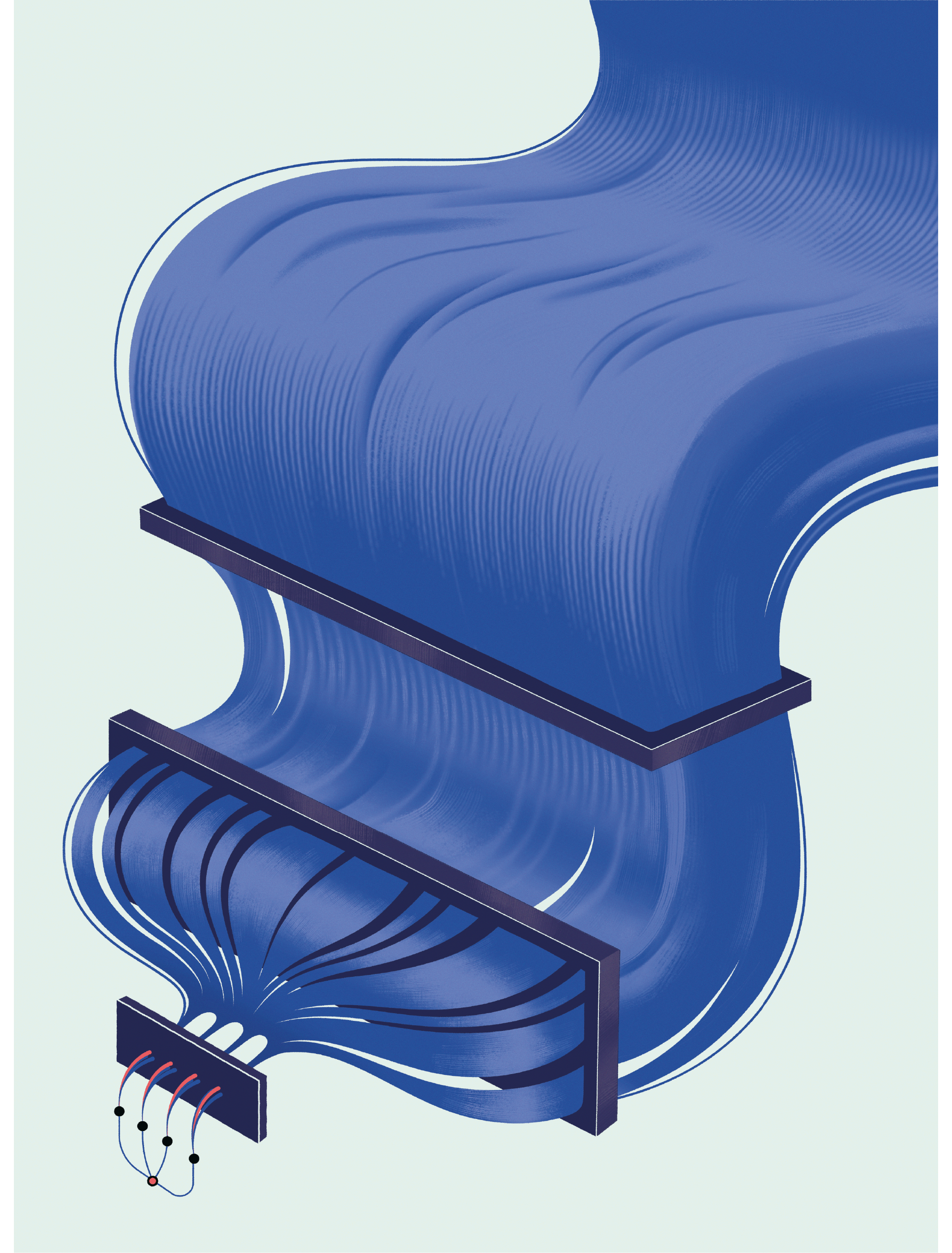
Illustration: Jakov Jakovljevic